今個人マシンとしてメインで使っている MacBook Air (OS X 10.9.2) で word2vec を動かしてみましたよ、というお話。
- word2vec - Tool for computing continuous distributed representations of words. - Google Project Hosting
https://code.google.com/p/word2vec/
- MacBook Air 13-inch (Mid 2013)
- Mac OS X 10.9.2 (Mavericks)
- 1.3GHzデュアルコアIntel Core i5
- メモリ 4GB
- Xcode が入っています。本記事での前提。
コードを svn でダウンロードします。
ディレクトリ移動して、おもむろに make。すると、distance.c, word-analogy.c, compute-accuracy.c にエラーが出ます。
コンパイラのエラーメッセージで指摘されますが、各ファイルの
で、改めて make すればOKです。
word2vecで使うデータを作成するためサンプルスクリプト「demo-word.sh」を実行。
(Macトラブル1) wget が入ってなかったのでエラー。wget をインストール。しかしいらなかった。
(Macトラブル2) 再度「demo-word.sh」を実行すると今度はターミナルアプリが落ちる。gzip がらみか。text8.zip をとってきて解凍する部分。
text8 は 0 行、17005207 ワード、100000000 バイトのテキストファイル。先頭はこんな感じ。
で、あらためて demo-word.sh を動かせば大丈夫。
5分くらいで構築完了。vectors.bin というデータができます。このデータを用いていろいろとやるのです。
入力を求められてるけど、ここは終了しておきます。
入力した単語・文に近い単語を出します。前節で作った vectors.bin を使います。
コマンド distance を vectors.bin を引数にして起動(前述「demo-word.sh」内でも同様のことしています)。
入力を求められます。で、まずは「tokyo」。上位10個だけ表示。出力フォーマットは一部加工済み(以降同様)。ふむふむ、東京に近いものが出てきていますね。
次は「sea of japan」の結果。国境がらみの海とか川とか、日本の海とか。Vardar はマケドニア、ギリシャを流れるヴァルダル川のこと。
単語じゃなくてフレーズを単位にやる話。ここで言うフレーズとは、「"Los Angeles" は "los" と "angeles" ではなく、一語で扱いたい!」という要求を満たすため、アンダーバーで繋げて los_angeles にしてしまうみたいな感じ。
「demo-phrases.sh」を実行します。
このシェルスクリプトはまず内部で word2phrase を動かし、入力 text8 を統計的な何やらでフレーズ認識し、text8-phrase として出力します。
text8-phrase の冒頭の部分を見てみると、text8 では"sans culottes" だった部分が "sans_culottes" とフレーズ認識され「1ワード」にされています。
次にシェルスクリプトはこの text8-phrase を分析して vectors-phrase.bin を作成します。前節の vectors.bin のフレーズ版です。
コマンド distance を vectors-phrase.bin を引数にして起動。
「los angeles」のフレーズ化である「los_angeles」で試してみます。ふむふむ。"san_francisco" や "san_diego" とか出てきていますね。(ちなみに vectors.bin で "los angeles" とやるとボロボロです)
「日本」に対しての「東京」にあたるものは、「中国」に対しては何? 「韓国」だと何? みたいなのを出します。
前節で作った vectors-phrase.bin を使います。
コマンド word-analogy を vectors-phrase.bin を引数にして起動します。
まずは、日本に対しての東京が韓国の何にあたるのか? うむ、ソウルですね、首都ですね。
以下、いろいろ。うまくいってるやつ。
「demo-classes.sh」を実行すると text8 を分析して単語をクラスタリング(グルーピング)してくれます。
結果は classes.sorted.txt というファイルに。同じ数字を持つ単語が同じクラス(グループ)になります。クラスタリングのアルゴリズムは K-means です(内部で呼ばれているコマンド word2vec の classes オプションで K を指定できます)。
フレーズでやるには、シェルスクリプト内の text8 を text8-phrase に変換すればOK。出力先ファイル名も classes-phrase.sorted.txt とかに変更しておきましょう。
- O'Reilly Japan - word2vecによる自然言語処理
http://www.oreilly.co.jp/books/9784873116839/
(電子書籍)
- Python - Perl + Java = ? はてなブログのデータとパソコン工房のPCを使って「word2vec」で遊んでみた (はてなニュース)
http://hatenanews.com/articles/201404/20050
(リンク集がある)
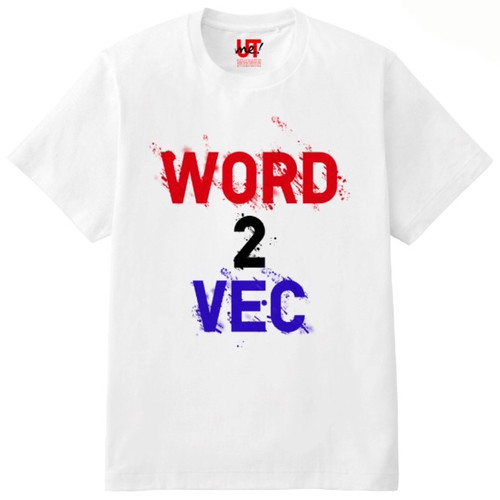
- word2vec - Tool for computing continuous distributed representations of words. - Google Project Hosting
https://code.google.com/p/word2vec/
マシン環境
- MacBook Air 13-inch (Mid 2013)
- Mac OS X 10.9.2 (Mavericks)
- 1.3GHzデュアルコアIntel Core i5
- メモリ 4GB
- Xcode が入っています。本記事での前提。
ビルド
コードを svn でダウンロードします。
svn checkout http://word2vec.googlecode.com/svn/trunk/
ディレクトリ移動して、おもむろに make。すると、distance.c, word-analogy.c, compute-accuracy.c にエラーが出ます。
コンパイラのエラーメッセージで指摘されますが、各ファイルの
を#include <malloc.h>
に置き換えます。#include <stdlib.h>
で、改めて make すればOKです。
サンプルデータ取得
word2vecで使うデータを作成するためサンプルスクリプト「demo-word.sh」を実行。
(Macトラブル1) wget が入ってなかったのでエラー。wget をインストール。しかしいらなかった。
(Macトラブル2) 再度「demo-word.sh」を実行すると今度はターミナルアプリが落ちる。gzip がらみか。text8.zip をとってきて解凍する部分。
要するに text8 というファイルができれば良いわけ。デフォルトの Apple gzip ではなく外部から取ってきた gzip でやればいいんだろうけど、面倒なので普通にコマンドラインで下記を実行して text8 を得る。wget http://mattmahoney.net/dc/text8.zip -O text8.gz gzip -d text8.gz -f
curl http://mattmahoney.net/dc/text8.zip -O text8.zip unzip text8.zip
text8 は 0 行、17005207 ワード、100000000 バイトのテキストファイル。先頭はこんな感じ。
% cut -c1-300 text8 | fold -w 60 anarchism originated as a term of abuse first used against early working class radicals including the diggers of the en glish revolution and the sans culottes of the french revolut ion whilst the term is still used in a pejorative way to des cribe any act that used violent means to destroy the organiz
で、あらためて demo-word.sh を動かせば大丈夫。
% ./demo-word.sh make: Nothing to be done for `all'. Starting training using file text8 Vocab size: 71290 Words in train file: 16718843 Alpha: 0.000121 Progress: 99.58% Words/thread/sec: 48.64k real 1m46.381s user 5m57.164s sys 0m1.418s Enter word or sentence (EXIT to break):
5分くらいで構築完了。vectors.bin というデータができます。このデータを用いていろいろとやるのです。
入力を求められてるけど、ここは終了しておきます。
距離 (単語)
入力した単語・文に近い単語を出します。前節で作った vectors.bin を使います。
コマンド distance を vectors.bin を引数にして起動(前述「demo-word.sh」内でも同様のことしています)。
% ./distance vectors.bin Enter word or sentence (EXIT to break):
入力を求められます。で、まずは「tokyo」。上位10個だけ表示。出力フォーマットは一部加工済み(以降同様)。ふむふむ、東京に近いものが出てきていますね。
% ./distance vectors.bin Enter word or sentence (EXIT to break): tokyo Word: tokyo Position in vocabulary: 4909 Word Cosine distance ----------------------------------- narita 0.662572 osaka 0.653032 incheon 0.607367 fukuoka 0.595367 beijing 0.571802 kansai 0.567351 seoul 0.564947 jiaotong 0.558960 sheremetyevo 0.558197 niigata 0.556976
次は「sea of japan」の結果。国境がらみの海とか川とか、日本の海とか。Vardar はマケドニア、ギリシャを流れるヴァルダル川のこと。
Enter word or sentence (EXIT to break): sea of japan Word: sea Position in vocabulary: 356 Word: of Position in vocabulary: 2 Word: japan Position in vocabulary: 582 Word Cosine distance -------------------------------- senkaku 0.570105 vardar 0.568383 seto 0.560345 dangrek 0.542683 endorheic 0.525477 caspian 0.520474 shikoku 0.518149 blantyre 0.516904 westeros 0.505320 honshu 0.504499
距離 (フレーズ)
単語じゃなくてフレーズを単位にやる話。ここで言うフレーズとは、「"Los Angeles" は "los" と "angeles" ではなく、一語で扱いたい!」という要求を満たすため、アンダーバーで繋げて los_angeles にしてしまうみたいな感じ。
「demo-phrases.sh」を実行します。
% ./demo-phrases.sh make: Nothing to be done for `all'. Starting training using file text8 Words processed: 17000K Vocab size: 4399K Vocab size (unigrams + bigrams): 2419827 Words in train file: 17005206 Words written: 17000K real 0m56.124s user 0m52.278s sys 0m2.000s Starting training using file text8-phrase Vocab size: 84069 Words in train file: 16307293 Alpha: 0.000117 Progress: 99.60% Words/thread/sec: 21.08k real 3m43.700s user 13m6.280s sys 0m2.559s Enter word or sentence (EXIT to break):
このシェルスクリプトはまず内部で word2phrase を動かし、入力 text8 を統計的な何やらでフレーズ認識し、text8-phrase として出力します。
text8-phrase の冒頭の部分を見てみると、text8 では"sans culottes" だった部分が "sans_culottes" とフレーズ認識され「1ワード」にされています。
% cut -c1-300 text8-phrase | fold -w 60 anarchism originated as a term of abuse first used against early working class radicals including the diggers of the en glish revolution and the sans_culottes of the french revolut ion whilst the term is still used in a pejorative way to des cribe any act that used violent means to destroy the organiz
次にシェルスクリプトはこの text8-phrase を分析して vectors-phrase.bin を作成します。前節の vectors.bin のフレーズ版です。
コマンド distance を vectors-phrase.bin を引数にして起動。
% ./distance vectors-phrase.bin Enter word or sentence (EXIT to break):
「los angeles」のフレーズ化である「los_angeles」で試してみます。ふむふむ。"san_francisco" や "san_diego" とか出てきていますね。(ちなみに vectors.bin で "los angeles" とやるとボロボロです)
Enter word or sentence (EXIT to break): los_angeles Word: los_angeles Position in vocabulary: 1680 Word Cosine distance ------------------------------------ california 0.625356 san_francisco 0.617207 san_diego 0.594875 taiko 0.555129 lakers 0.551306 san_jose 0.550566 oakland 0.546525 santa_monica 0.535825 beverly_hills 0.535591
アナロジー
「日本」に対しての「東京」にあたるものは、「中国」に対しては何? 「韓国」だと何? みたいなのを出します。
前節で作った vectors-phrase.bin を使います。
コマンド word-analogy を vectors-phrase.bin を引数にして起動します。
% ./word-analogy vectors-phrase.bin Enter three words (EXIT to break):
まずは、日本に対しての東京が韓国の何にあたるのか? うむ、ソウルですね、首都ですね。
Enter three words (EXIT to break): japan tokyo korea ord: japan Position in vocabulary: 547 Word: tokyo Position in vocabulary: 4715 Word: korea Position in vocabulary: 2559 Word Distance ---------------------------------------- seoul 0.485544 south_korea 0.438259 paekche 0.427070 chungcheong_south 0.418033 osaka 0.400935
以下、いろいろ。うまくいってるやつ。
Enter three words (EXIT to break): new_york los_angeles tokyo ... Word Distance -------------------------------- osaka 0.483591 taipei 0.479719 kaohsiung 0.446331 seoul 0.433824 akihabara 0.428942 Enter three words (EXIT to break): bread eat beer ... Word Distance ------------------------------------- drink 0.416754 custard 0.400807 keg 0.396400 eating 0.385646 milk_chocolate 0.378704 Enter three words (EXIT to break): cat lion dog ... Word Distance --------------------------------------- wolf 0.375175 belgian_shepherd 0.370185 keeshond 0.367813 hound 0.367517 bear 0.360686
クラスタリング
「demo-classes.sh」を実行すると text8 を分析して単語をクラスタリング(グルーピング)してくれます。
% ./demo-classes.sh make: Nothing to be done for `all'. Starting training using file text8 Vocab size: 71290 Words in train file: 16718843 Alpha: 0.000121 Progress: 99.58% Words/thread/sec: 48.55k real 2m32.658s user 6m42.357s sys 0m1.459s The word classes were saved to file classes.sorted.txt
結果は classes.sorted.txt というファイルに。同じ数字を持つ単語が同じクラス(グループ)になります。クラスタリングのアルゴリズムは K-means です(内部で呼ばれているコマンド word2vec の classes オプションで K を指定できます)。
formerly 493 fort 493 founded 493 fountain 493 francisco 493 frankfurt 493 fredericton 493 freeway 493 frontage 493 galleries 493 gallery 493
フレーズでやるには、シェルスクリプト内の text8 を text8-phrase に変換すればOK。出力先ファイル名も classes-phrase.sorted.txt とかに変更しておきましょう。
salt_lake 478 sam_houston 478 samora 478 san_antonio 478 san_bernardino 478 san_diego 478 san_fernando 478 san_francisco 478 san_jacinto 478 san_joaquin 478 san_jose 478 san_juan 478
参考文献
- O'Reilly Japan - word2vecによる自然言語処理
http://www.oreilly.co.jp/books/9784873116839/
(電子書籍)
- Python - Perl + Java = ? はてなブログのデータとパソコン工房のPCを使って「word2vec」で遊んでみた (はてなニュース)
http://hatenanews.com/articles/201404/20050
(リンク集がある)
この記事に言及しているこのブログ内の記事